Source camera idnetification, deep fake detection
ディジタルカメラ、スマートフォン、スキャナ等の撮影機器で撮影した画像中にはぱっと見ただけではわからないですが、実は非常に多くの情報が隠されています。Photo Response Non-Uniformity (PRNU)ノイズもその中のひとつです。 There is a great deal of information hidden in images captured by digital cameras, smartphones, scanners, and other imaging devices, including Photo Response Non-Uniformity (PRNU) noise.
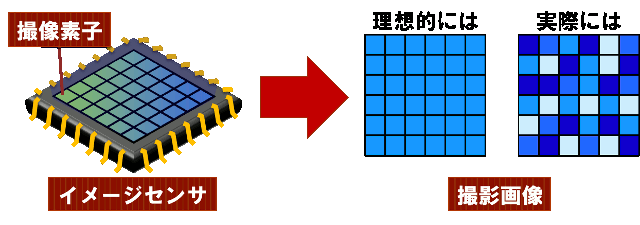
図1:Photo Response Non-Uniformityノイズ / Photo Response Non-Uniformity noise
一般的なディジタルカメラは図1のように各画素に対応してひとつの撮像素子を持っており、 撮像素子に入射した光を電圧に変換して画素値を求めています。 これらの撮像素子は全て同じ感度であること望まれますが、実際には製造ばらつきでこの撮像素子の感度は画素毎に異なります。 結果として、画像中にPRNUノイズと呼ばれる微弱なパターンノイズが生じてしまうのです。 このパターンノイズはカメラ毎に異なるので、カメラの指紋と見なせます。 つまり、画像中のPRNUノイズを比べることで、その画像をどのカメラ、どのスマートフォン、どのスキャナで撮影したかが特定できるのです。 この技術は盗撮,違法コピー等の犯罪の抑止力として期待されています。 As shown in Fig. 1, a typical digital camera has an image sensor for each pixel, and the light incident on the image sensor is converted to a voltage to obtain the pixel value. It is hoped that all of these image sensors have the same sensitivity, but in reality, the sensitivity of these image sensors varies from pixel to pixel due to manufacturing variations. As a result, a faint pattern noise called PRNU noise is generated in the image. This pattern noise varies from camera to camera and can be regarded as a fingerprint of the camera. In other words, by comparing the PRNU noise in the image, it is possible to determine which camera, smartphone, or scanner took the image. This technology is expected to be a deterrent to crimes such as spy photography and piracy.
図2:改竄部位の検出(左:改竄画像、右:改竄部位の正解データ)
Figure 2: Detection of forgery area (left: forgery image, right: correct data for forgery area)
また、図2のような改竄画像では、改竄された部分のノイズが本来のPRNUノイズと変わってしまうため、 改竄部位の検出への応用も可能です。 In addition, in a forgery image such as the one in Figure 2, the noise in the forgery area changes from the original PRNU noise. It can also be applied to the detection of forgery areas.
PRNUノイズ以外にも画像中には様々なパターンノイズが存在します。これらのパターンノイズを活用することで,ディープフェイク検出に貢献することを目指しています。 In addition to PRNU noise, there are various other pattern noises in the image. By utilizing these pattern noises, we aim to contribute to deep fake detection.